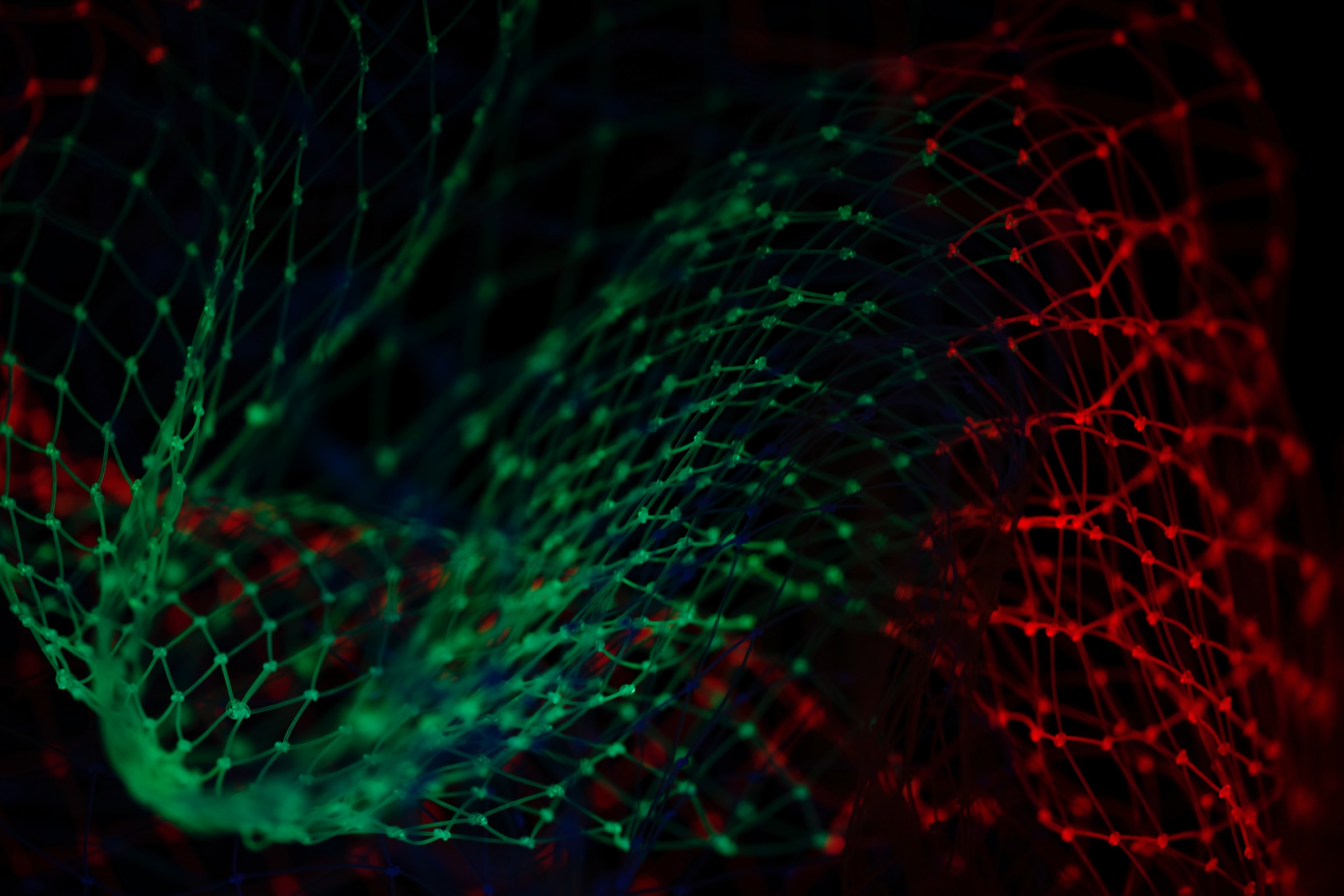
Top 10 emerging AI and ML uses in data centres
In this week’s round-up, we share the top 10 ways AI and ML technologies are transforming the world’s data centres, driving efficiencies and sustainability
Across the world’s industries, AI and ML are enabling a huge degree of transformation.
These technologies – in their many forms – are being utilised to drive improvements in operational efficiency, sustainability and capacity management, just to name a handful.
In data centres, AI and ML solutions are being deployed at pace, in order to equip sites with the solutions necessary to manage the world’s rising data demands, alongside ever-ambitious sustainability targets.
So, in this week’s top 10, we outline the leading AI and ML use cases that are emerging in the data centre industry.
10) Sustainability aids
Through AI and ML models, data centres can pinpoint precisely what areas are most impacting their power usage effectiveness (PUE) and address them.
Alongside this, the models can identify the optimum conditions and WUE for a data centre.
In short, they can identify a way of operating that balances performance and sustainability in the most effective way possible.
As a result, these technologies are a highly efficient and impactful way for data centres to improve their sustainability standards.
And, with consumers prioritising sustainable partners more than ever before, this investment has never been so pressing.
9) Natural Language Processing tools
Through Natural Language Processing (NLP) tools, mission-critical operations can be simplified, at a remarkable speed.
These tools are increasingly being deployed in a wide range of mission-critical processes and enterprise solutions, including:
- Text summarisation
- Machine translation
- Chatbots
- Detecting spam or phishing emails
8) Anomaly detection
AI and ML tools are remarkably proficient at identifying patterns and pinpointing anomalies.
As a result, they are an invaluable aid to data processing and data management and can spot anomalies or perform root cause analysis far, far faster than the human brain can.
7) Monitoring and debugging
Tools like TensorBoard, Weights & Biases and Neptune are increasingly being used by IT teams to perform monitoring and debugging.
As with anomaly detection, AI and ML tools can perform these tasks significantly faster and more accurately than is possible for humans.
6) Asset performance management
Asset performance management involves capturing, integrating and analysing data, so that a data centre’s physical assets can be used as effectively as possible.
AI and ML models can not only increase the lifespan of data centre assets (by identifying potential flaws in their usage), but they can also recommend predictive maintenance schedules, and alert managers to fluctuations in equipments’ normal operating conditions.
5) Maximising uptime
Building on the value of AI and ML tools in asset performance management, by ensuring assets are used effectively and protected against damage, these tools are proving invaluable in maximising data centre uptime.
Reliability is the crux of a data centre’s reputation. And, through predictive maintenance, equipment preservation and advance flaw warnings, AI and ML tools significantly reduce the likelihood of a data centre outage.
4) Capacity planning and management
Many of the world’s data centres are in a state of constant expansion.
As a result, the industry stands to make major gains from leveraging AI and ML technologies that can perform capacity planning and management.
Not only are these technologies enabling data centres to upscale seamlessly, but they are helping sites to do so while keeping waste and costs to the minimum.
3) Customer relationship management
AI and ML are widely associated with NLP chatbots. But, what is much less commonly known about these tools is their capacity to improve the broader customer experience with a company.
For example, AI and ML can be used to identify customers that are at a high risk of leaving, and alert teams, while recommending suggestions to rebuild those customer connections. Then, teams can proactively offer more targeted support, to restore the situation.
2) Cybersecurity
Data leaks and cyberattacks pose a huge threat to data centres.
But, using specialist AI and ML models, providers can implement stronger cybersecurity protocols, identify any weak areas in their system, and spot any suspicious activity, before it poses a larger threat.
1) Improve workflow productivity
By utilising previous learnings, and implementing tailored solutions based on this, AI and ML tools can help data centres fix incidents far more efficiently.
Plus, in all of the ways listed above, these platforms can open up extensive opportunities for improved efficiencies, in everything from the assets on-site, to the way customer experience is handled.