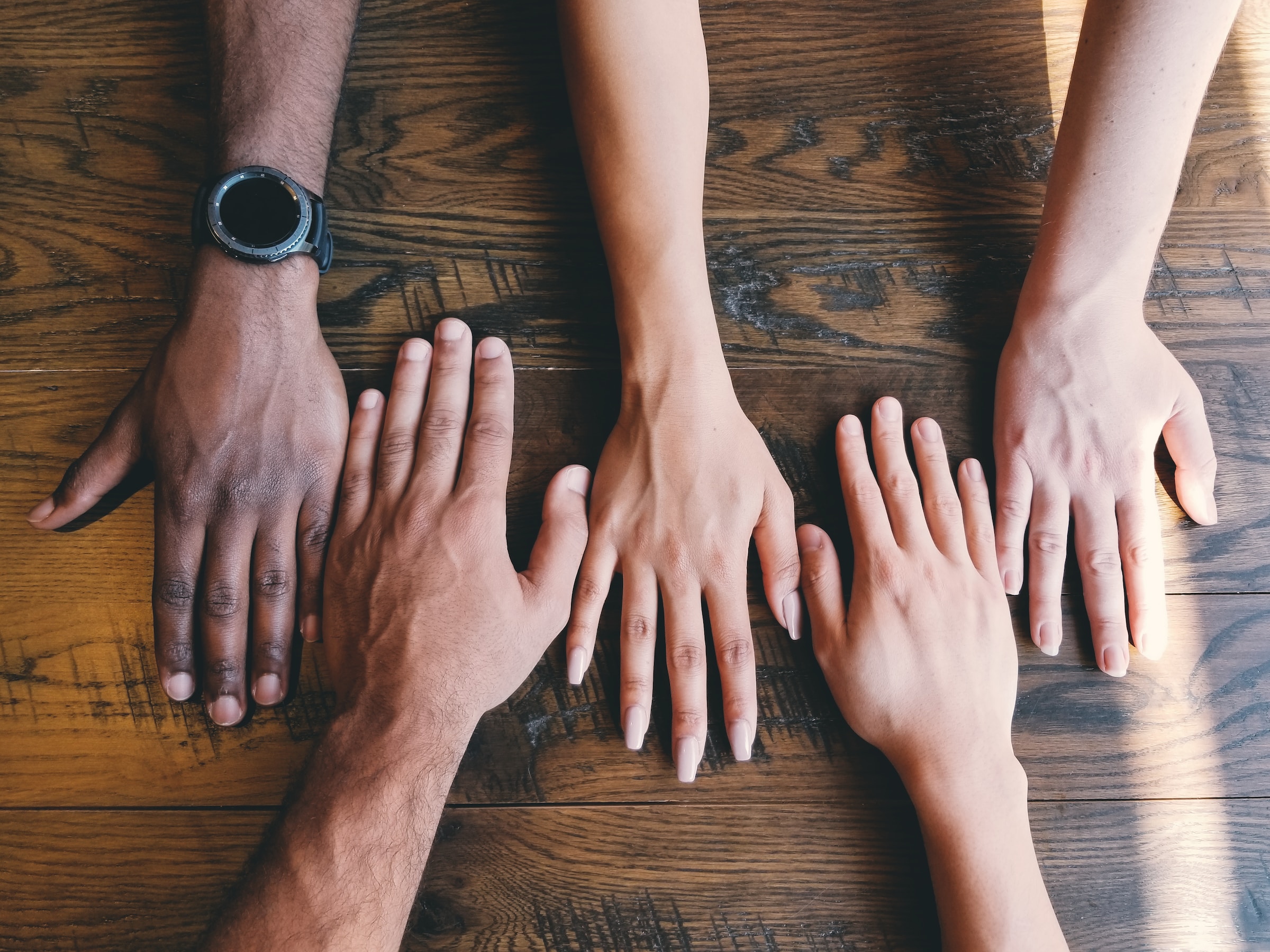
Study highlights impact of demographics on AI training
A study conducted in collaboration between Prolific, Potato, and the University of Michigan has shed light on the significant influence of annotator demographics on the development and training of AI models.
The study delved into the impact of age, race, and education on AI model training data—highlighting the potential dangers of biases becoming ingrained within AI systems.
“Systems like ChatGPT are increasingly used by people for everyday tasks,” explains assistant professor David Jurgens from the University of Michigan School of Information.
“But on whose values are we instilling in the trained model? If we keep taking a representative sample without accounting for differences, we continue marginalising certain groups of people.”
Machine learning and AI systems increasingly rely on human annotation to train their models effectively. This process, often referred to as ‘Human-in-the-loop’ or Reinforcement Learning from Human Feedback (RLHF), involves individuals reviewing and categorising language model outputs to refine their performance.
One of the most striking findings of the study is the influence of demographics on labelling offensiveness.
The research found that different racial groups had varying perceptions of offensiveness in online comments. For instance, Black participants tended to rate comments as more offensive compared to other racial groups. Age also played a role, as participants aged 60 or over were more likely to label comments as offensive than younger participants.
The study involved analysing 45,000 annotations from 1,484 annotators and covered a wide array of tasks, including offensiveness detection, question answering, and politeness. It revealed that demographic factors continue to impact even objective tasks like question answering. Notably, accuracy in answering questions was affected by factors like race and age, reflecting disparities in education and opportunities.
Politeness, a significant factor in interpersonal communication, was also impacted by demographics.
Women tended to judge messages as less polite than men, while older participants were more likely to assign higher politeness ratings. Additionally, participants with higher education levels often assigned lower politeness ratings and differences were observed between racial groups and Asian participants.
Phelim Bradley, CEO and co-founder of Prolific, said:
“Artificial intelligence will touch all aspects of society and there is a real danger that existing biases will get baked into these systems.
This research is very clear: who annotates your data matters.
Anyone who is building and training AI systems must make sure that the people they use are nationally representative across age, gender, and race or bias will simply breed more bias.”
As AI systems become more integrated into everyday tasks, the research underscores the imperative of addressing biases at the early stages of model development to avoid exacerbating existing biases and toxicity.
You can find a full copy of the paper here (PDF)
(Photo by Clay Banks on Unsplash)
See also: Error-prone facial recognition leads to another wrongful arrest
Want to learn more about AI and big data from industry leaders? Check out AI & Big Data Expo taking place in Amsterdam, California, and London. The comprehensive event is co-located with Digital Transformation Week.
Explore other upcoming enterprise technology events and webinars powered by TechForge here.