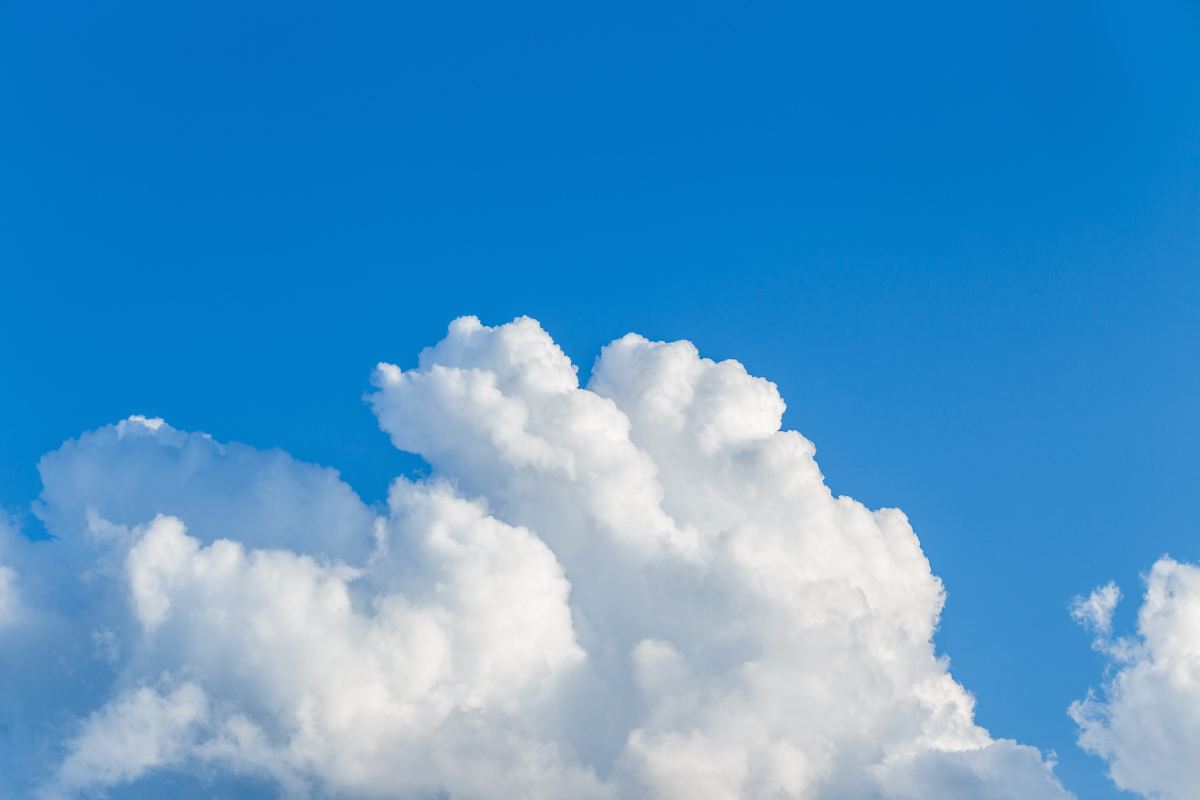
Deepset secures $30M to expand its LLM-focused MLOps offerings
Deepset, a platform for building enterprise apps powered by large language models akin to ChatGPT, today announced that it raised $30 million in a funding round led by Balderton Capital with participation from GV and Harpoon Ventures.
The proceeds will be put toward expanding Deepset’s products and services and growing its team from around 50 people to 70 to 75 by the end of the year, co-founder and CEO Milos Rusic says.
“In many organizations, data science teams are still the default option for ‘all things AI.’ In reality, a lot of data science teams are restructuring, relearning and reshaping their habits to match the growing demands of the product teams and the end-users in the enterprise,” Rusic told TechCrunch in an email interview. “The industry is shifting from AI labs to AI factories — it’s not anymore about tinkering around, it’s about shipping successful products and value.”
Rusic’s not wrong in implying that data science teams are overworked and overburdened. According to one recent poll, the vast majority of data engineers — the data scientists who prep data for analytics tools — are experiencing burnout, likely to leave their current company for another within 12 months and considering quitting the industry altogether.
The unfortunate state of affairs is likely contributing to challenges around AI development within the enterprise. A 2022 Gartner poll found that only around half of AI projects make the leap from pilot to production and that 53% of machine learning models are never deployed.
Rusic co-launched Deepset with Malte Pietsch and Timo Möller in 2018, bootstrapping the business by training custom natural language processing models for enterprises. The three co-founders closely followed the Transformer AI model architecture developed by Google in 2017, which would go on to form the basis of sophisticated LLMs like ChatGPT and GPT-4.
In 2019, Rusic, Pietsch and Möller released Haystack, an open source framework to build NLP back-end services with Transformers and other LLM architectures. The goal was to provide a collection of tools for software engineers to quickly create LLM-driven applications, Rusic says — particularly applications covering a specific use case, like helping legal teams search across case files.
But Deepset’s ambitions eventually outgrew Haystack.
Last year, the startup debuted Deepset Cloud, which Rusic describes as an “enterprise LLM platform for AI teams.” Deepset Cloud extends Haystack by providing a platform where customers can try out different LLMs, embed those LLMs into applications, deploy the applications and LLMs to end users, and perform analyses of the LLMs’ accuracy while continuously monitoring their performance.
Deepset Cloud also includes components for measuring and mitigating common issues with LLMs, like hallucination. Hallucination, which plagues even the best LLMs today, causes models to make up false information or facts that aren’t based on real events or data.
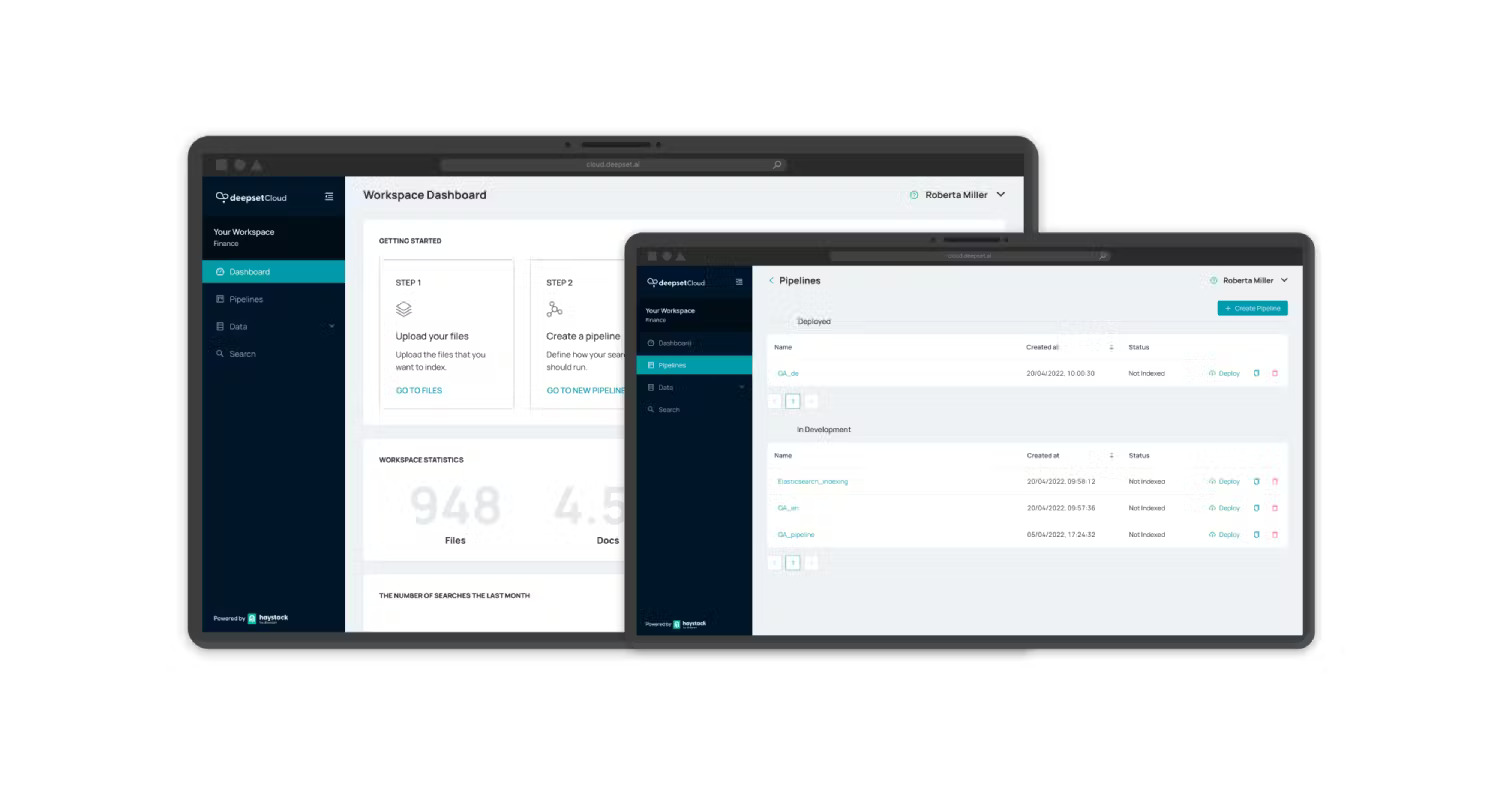
A screenshot of Deepset Cloud, Deepset’s new MLOps platform. Image Credits: Deepset Cloud
“Deepset Cloud leverages the open source Haystack technology very heavily — the pipeline architecture, the core components, datastores, integrations and so on,” Rusic explained. “Our platform delivers all the building blocks to avoid doing any ‘undifferentiated heavy-lifting’ and enables developers to focus on shipping NLP back-end services — API-driven, easily composable, easily embeddable and easily monitored.”
Deepset, which has raised a total of $46 million in funding to date, sees vendors competing in the MLOps space as its main rivals. MLOps attempts to streamline the process of building and managing machine learning models by providing tools to address each individual stage of a model’s life cycle.
Besides incumbents such as AWS, Azure and Google Cloud, a growing raft of startups provide MLOps products, platforms and services to enterprise clients. There’s Seldon, which recently raised $20 million; Galileo; McKinsey-owned Iguazio; Diveplane; Arize; and Tecton, to name a few.
Allied Market Research predicts that the sector for MLOps will reach $23.1 billion by 2031, up from around $1 billion in 2021. No doubt, the addressable market’s sheer size will continue to attract new entrants.
But Rusic points to Deepset’s expansion as evidence that it’s standing out from the crowd. The startup has “hundreds” of customer pipelines running on its platform, including workloads for Siemens and Airbus. Legal publishing house Manz tapped Deepset to launch an internal AI-powered tool that helps to surface court documents, related precedents and more. Airbus, meanwhile, is using Haystack to build apps that recommend aircraft operations guidelines to pilots in the cockpit.
“It’s often 10x faster to repeatedly build production-ready NLP and LLM services with Deepset Cloud as opposed to hiring, training and managing a dedicated team for robust back-end application development,” Rusic said. “Deepset Cloud allows customers to use various LLMs simultaneously, combining them in the application architecture to avoid vendor lock-in and mitigating data privacy and model sovereignty issues.”